In recent months there has been a little recurring and sometimes redundant music: AI does not exist ( Luc Julia in February 2019), AI startups do not do AI ( MMC Venture study in March 2019), AI hides a modern slavery of the labeling of data ( Cash Investigation report in September 2019 ), AI does not work without this or that (data and skills), AI projects do not succeed in companies , l ‘ AI is a scam and AI is above all dangerous, for example, the ban on facial recognition in certain cities (in San Francisco in May 2019 and in Portland in December 2019).
We could extrapolate all this by predicting that AI would be almost on a slippery slope, with plenty of room to enter a third winter of AI after those of the 1970s and 1990s.
To find out if AI was still in tune with the times, I took a look at Google Trends . We can see there on the key terms of the field that the interest on the subject has grown between 2016 and 2018 and that we have since reached a certain plateau. It is not yet half a Gaussian, but it could eventually become so.

But as we will see, the AI is not yet on a slippery slope … down! Or, we should consider that smartphones are in decline since their sales have been decreasing for two years in the world (due to a high rate of equipment)!
AI B2Bization
The potential disenchantment with AI can manifest itself in companies that are starting to accumulate feedback. So even if it’s still well hidden, chatbots made in a hurry do not work that well. Only those which have been created with strong means and clear objectives give good results. AI projects would not succeed in half of the cases and in addition, it would be difficult to measure their return on investment . These empirical studies are interesting but they generally lack a comparative perspective compared to usual digital projects. What if these failures were the norm and not the exception of AI? And on the other hand, AI projects that are well documented in case studies are very rare. Too bad.
At the same time, all businesses are mobilizing to integrate AI into their activities, as they have done in the past with microcomputing, Internet or mobility. Health, education, HR, agriculture, construction, media, banking and insurance, telecoms, everything goes! And some even find it going too fast !
In practice, these organizations and trades are rather spoiled for choice in the projects to be launched which integrate AI bricks. Most often, these are building blocks of image, language, noise or structured data. We are witnessing a “B2Bization” of AI after a flood in the general public with the management of the photo in your smartphones (all that for taking selfies…) and voice control ( Alexa , Google Assistant, Siri,…) .
Current AI, based mainly on deep learning, is becoming commonplace. By the way, the more you use it, the more you get out of the phantasmagorical and mystical visions of an AI that would exceed Man after having reached and exceeded the threshold of his intelligence. AI is losing its magic side.
Democratization of AI
AI is also democratizing thanks to the growing number of tools for its creation, and which are of an increasingly high level. After Google , Microsoft , DataRobot and Prevision.io and so many others, it was Amazon ‘s turn to launch SageMaker Autopilot in AutoML or AutoAI, this category of software tools that can automatically determine the models of machine learning and deep learning to exploit large volumes of data, in particular for classification, identification of correlations between observation data and for forecasting.
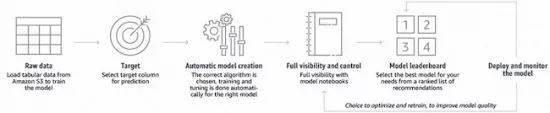
AI gradually dissolves in all software that manages data, which makes the world! There is still a lot to be invented, especially in language processing and collaborative work. Some of these novelties may therefore be slow to emerge when they are aimed at market niches. This explains the variability in the density of innovations between sectors of activity.
The quest for data remains an issue that preoccupies quite a few organizations and even tends to block them in their creativity around AI. They have over-swallowed the message that only GAFAs have adequate data volumes for AI. I had tried to put this fear into perspective in GAFA, companies and AI data published in July 2019. The trend is even towards the creation of deep learning models which require more reasonable volumes of data to train .
In healthcare, the branch of AI that works best is image recognition. The announcements are similar and follow one another of AI performances which recognize and characterize pathologies, often cancerous, in X-rays, MRIs and other biopsies. They are just now starting to be deployed! It is therefore rather the end of the beginning than the beginning of the end of this History.
AI hardware advancements
Algorithmic progress continues to advance in deep learning, but also in hardware, both for servers and training and for embedded, edge and endpoint computing (AI in or near connected objects). New extraordinary processors appeared in 2019 like that of Cerebras . Pulse neural processors (IBM TrueNorth, Intel Loihi) are also developing in parallel. Without counting the original approaches of the French LightOn and AnotherBrain that I would have the opportunity in 2020 to analyze in detail.
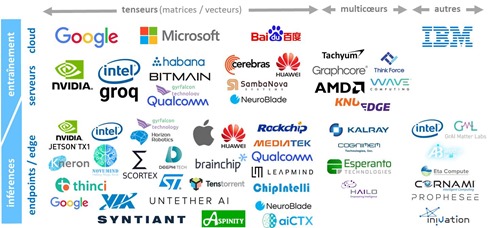
Qualcomm announced this week to further double the inference power of its latest smartphone chipset the Snapdragon 865, with a power of 14 TOPS (tera-operations in integers per second). In 2020, we could see Nvidia changing the game again with the successor to the legendary V100 GPU used massively in data centers and for training deep learning models.
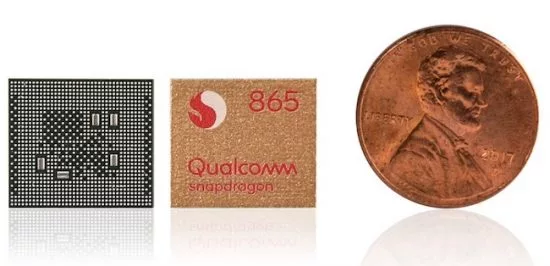
These technological advances make it possible to partially respond to an objection to AI, which is its carbon footprint. It is not negligible but is drowning for the moment in the general consumption of digital tools of which it is only one aspect.
Impact of AI on jobs?
This anticipation of a fall in AI coexists with that, completely opposite, of jobs that would be inexorably replaced by AI, to the point that almost all human tasks would be threatened, including the professions of researchers. As we like symbols, that of the premature retirement of the champion of Go Lee Sedol(36 years old) made an impression. He takes it sooner than a professional soccer player! And this is not because of the youngest, but because he judges that he can no longer fight against AI. Fortunately, the profession of professional Go player is not common, the impact of this news is completely anecdotal and unimportant on global employment. Are Go players covered by a special pension plan? The announcement has the same effect on me as if we decided to stop learning to count at school because of spreadsheets, or to read and write because of the deployment of voice control! That being said, several states in the USA decided to stop learning cursive handwriting a few years ago.
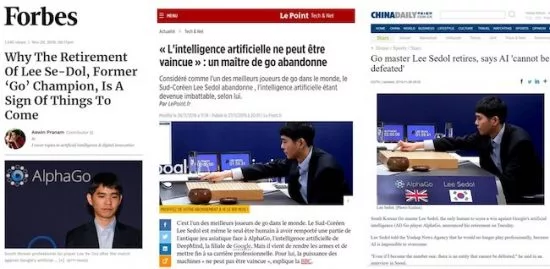
In Why The Retirement Of Lee Se-Dol, Former ‘Go’ Champion, Is A Sign Of Things To Come (Forbes, November 2019), Aswin Pranam urges us in any case to revisit our professional activities: ” we need to reassess the beliefs , values, attributes, and skills that make us who we are. Otherwise, we fall victim to hard questions about meaning when the next AI wave arrives ” . Good question. Long answers, to be found task by task and profession by profession!
If companies still advertise layoffs, it’s rarely because of AI, at least not directly. When banks lay off, it is because of the transition from an agency job to online banking. Admittedly, it can rely in part on chatbots like Orange Bank does, but it is not enough. IBM would have reduced the size of its HR thanks to AI, but that concerns few people. And if they dismiss, it will be mainly for reasons of lack of competitiveness compared to other players in the IT services market. We were talking a few years ago about the unfortunate fate of lawyers. For now, the advent of AI in law firms does not seem to have generated human victims. The consequences of any automation are always less direct than it seems. It can thus help widen a market by making certain services more affordable. It’s pretty tricky to predict.
On the other hand, we are not immune to another phenomenon: today, the technical skills around AI are lacking. Companies have trouble recruiting. In all countries, a large number of higher education programs are implemented to compensate for this. It is not impossible that in a few years, they generate profiles against the current of demand. We already knew this in the 1990s and 2000s.
Ethical AI
Initiatives and conferences on ethical AI abound. Latest initiative to date, UNESCO which launched in December 2019 the creation of an “ international normative instrument on the ethics of artificial intelligence” in November 2019. The international organization will create a group of experts who will give a notice by September 2020. The text will then be adopted in 2021. It is likely that this text will closely resemble other AI texts and ethics charters such as the Montreal Declaration of late 2018.
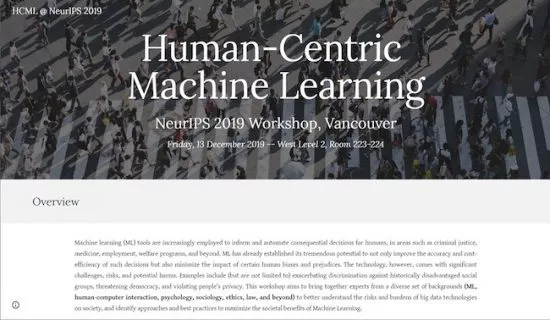
We are worried about killer robots (Stuart Russel) or fake news generated by generative neural networks. But that does not prevent moving forward on the rest. Too much AI ethics won’t kill AI. Rather, it will help make it acceptable and develop it. From there to do “ Human centric machine learning ” as proposed by the NeurIPS 2019 conference? The ethics washing AI lurks around the corner!
Beyond the semantic quarrel of AI
The wave of semantic quarrel over what AI is is far from over. I remind you of my extensive definition: AI brings together all the techniques that aim to emulate, imitate or extend, generally in pieces, components of human intelligence. This has been found in the scientific literature on AI since the 1950s.
This technically includes a very broad scientific field which has more than six decades of experience with the tools of logical reasoning, machine learning (machine learning, neural networks and deep learning) and multi-agent systems. Some AI bricks are fairly anthropomorphic in nature such as language processing or vision and others are already far beyond human capacity when it comes to crunchering very large volumes of data.
The battle between connectionists and symbolists seems to have been recently relaunched by Yann Le Cun, at least via a tweet which generated a sharp reaction from Gary Marcus, an American scientist and entrepreneur in AI who wishes to bring symbolic and connectionist methods closer to l ‘IA. In any case, the too direct association between AI and machine learning and deep learning is very simplistic.
When in To Understand The Future of AI, Study Its Past, Rob Toews writes that “ Neural networks do not develop semantic models about their environment; they cannot reason or think abstractly; they do not have any meaningful understanding of their inputs and outputs. Because neural networks’ inner workings are not semantically grounded, they are writable to humans “ , it confuses the methods currently used in neural networks (convolutional, with memory) and their general principle that nothing should theoretically prevent the manipulation of symbols, like the human brain. The latter, until proven otherwise, is also a (large) neural network!
The separation between symbolic AIs and connectionists should focus more on comparing the effectiveness of different methods to obtain an equivalent result. We can manipulate graphs and ontologies with symbolic and logical AI as well as with neural networks. All this explains the interest of continuing research on the functioning of the human brain, which is based on different techniques such as functional MRI, which is used to detect active areas of the brain according to the tasks it performs. It is a way to avoid ending up in the dead end that some people feel in research on deep learning, like Jérôme Pesenti of Facebook , who is less optimistic than Yann Le Cun on this point (he is his N + 1 at FAIR).
Observation and simulation
One of the serious challenges to AI may come from quantum computing. Not because we will be able to execute certain machine learning algorithms more efficiently on a quantum computer than on a conventional computer (it will be possible), but rather because these will allow to solve certain problems to go out of the logic of observation deep learning connectionists. The best example is that of the evaluation of the folding of proteins on themselves.
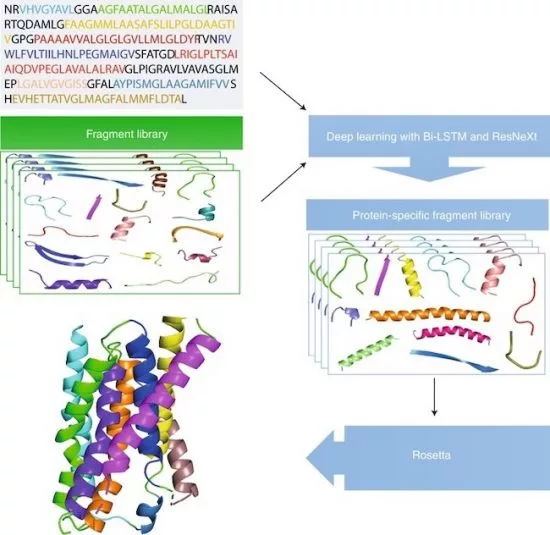
With deep learning, embodied since the end of 2018 by the AlphaFold method of DeepMind , we evaluate this folding by exploiting a neural network trained with the knowledge that we have of the folding of known proteins. With quantum computation, we can directly use the laws of quantum physics to determine this aliasing. It is still questionable but it allows us to understand the difference: between probabilistic evaluation and direct calculation.
When the laws of Newtonian or quantum physics can be implemented in computation, one can do without the probabilistic approach. Especially insofar as it is constrained by our knowledge of the existing and can hardly get out of it unlike simulation. The key is the invention of new therapies. The deadline: perfectly impossible to determine and probably several decades.
In any case, AI like quantum computing will not create a perfectly deterministic world and that’s good.
AI Startups
The other indicator to use to analyze the waves is that of investment in startups. It seems for now that 2019 is still a good year in growth for AI startups and on both sides of the Atlantic as well as in China.
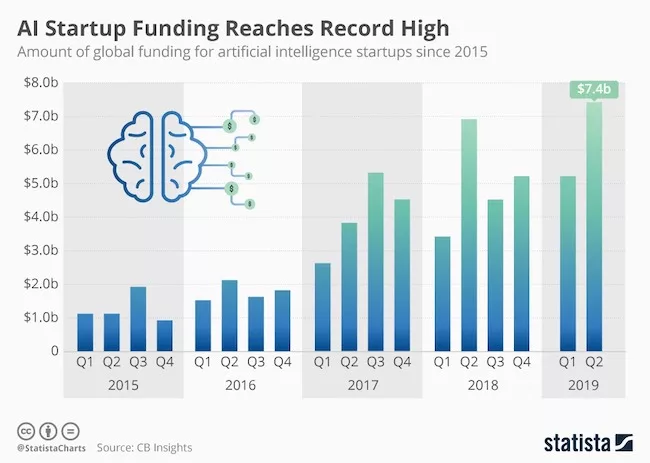
Even if it will probably be a year dominated by “series C”, these rounds of financing of tens or even hundreds of millions of dollars, like this last round realized by Dataiku (2013, 146 million dollars) with Google Ventures. A French startup that understood the game and now presents itself as a New York startup! Seed investments in AI startups, on the other hand, tend to calm down.
We will see what 2020 will give. It is possible that we are witnessing a decrease in the number of AI startups funded. This could be explained by several factors: a calm in the funding of startups in general, the trivialization of AI among all startups that make software, or the emergence of larger technological waves even if we do not still sees neither the color nor the mass (Blockchain, VR / AR).
Geopolitics
Another signal that the AI is still very much alive, the continuation of the geopolitical battle that surrounds it. American researchers in the field continue to lobby for the creation of an Artificial Intelligence Initiative Act , which is still being debated in Congress (in December 2019). The Russians who wake up and have just formalized their own AI plan, with a fairly strong military connotation . As for the Chinese who have trumpeted their goal of dominating the world of AI with their research and their entrepreneurs, they would not invest that much in the field. This also gives grain to grind for Americans who use the Chinese scarecrow to obtain credits as was the case for the $ 1.25 billionNational Quantum Initiative Act validated in December 2018.
In short, the wave of AI does not seem to be exhausted. Sooner or later it will merge with all of the digital technologies and will become an ordinary component. Finally, AI is just software with data!
The debate remains open, however! It’s your turn!